The transformation in retail that started in 2020 is not about new trends. Rather, it is defined by mass adoption of services that were previously offered by only selected retailers. The new purchase journey now takes four distinct paths: 1) drive and purchase – travel to a brick-and-mortar store; 2) click and pick – purchased online and pickup at the store; 3) click and deliver – purchase online and have the item delivered; and 4) subscribe and forget – subscribe online and have the item delivered a regular interval (CPG brands). Consumers have adapted to these services and clearly see trade-off between time/effort and money. The retailers that will come out on top will be those that can offer the most frictionless purchase journeys.
Notebook Thoughts: Machine Learning for Dummies
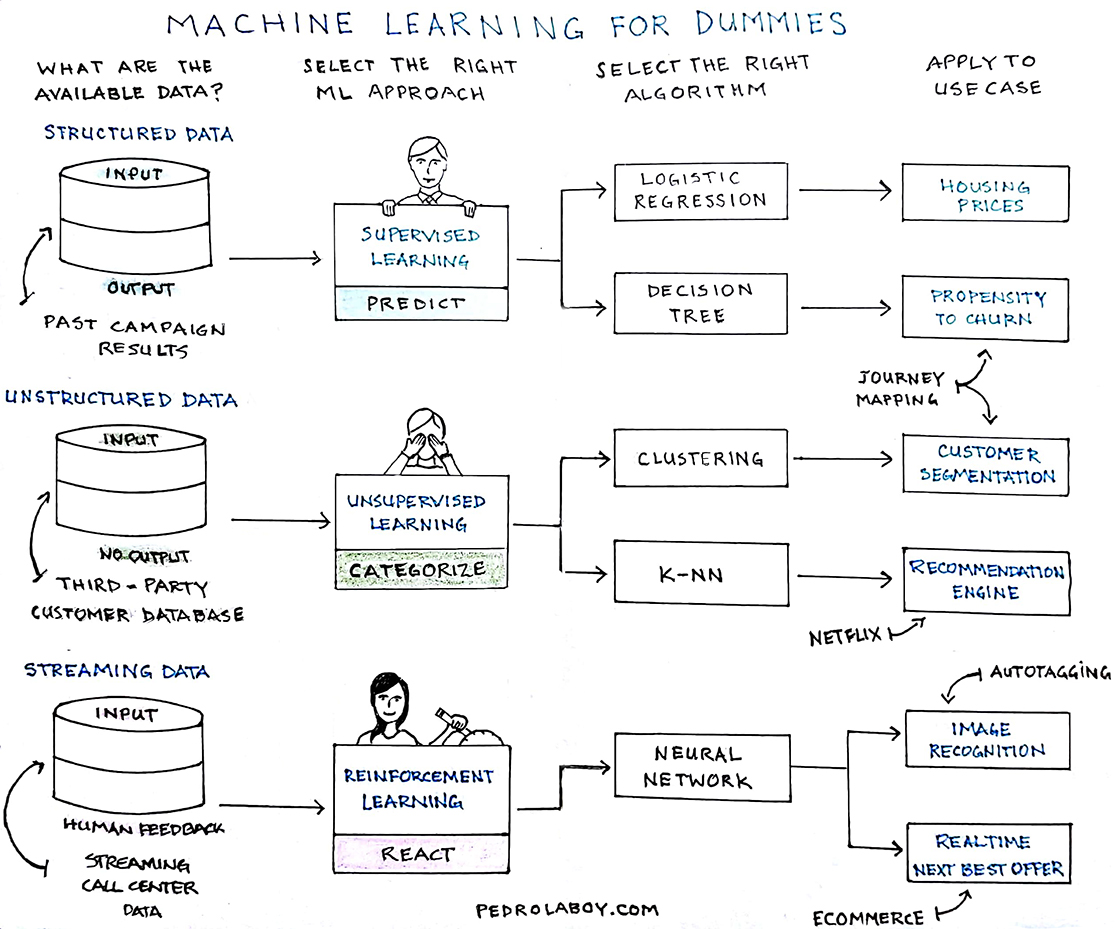
Machine learning is an evolving and complex science. If one takes into account all possible scenarios, dependencies and models, it would be impossible to sketch an all-encompassing explanation. So I will focus on what the non-data scientist should know about machine learning. That is, they should focus on understanding available data, machine learning types, algorithm models and business applications.
1. First, determine if what is the nature of the available data. Do you have historical campaign data with historical results or an unstructured database of customer records? Or are you trying to make real-time decisions on streaming data?
2. The data available–and use case–will determine the appropriate machine learning approach. There are three major types: supervised, unsupervised and reinforcement learning. a) Supervised Learning allows to you to predict an outcome based on input and output data (e.g. churn). b) Unsupervised Learning allows to you categorize outcomes based on input data (e.g. segmentation). c) Reinforcement Learning allows you to react to an environment (e.g. driverless car).
3. Each machine learning type will use a number of algorithm. There are hundreds of variations. Supervised Learning typically uses regression or classification algorithms. Unsupervised Learning uses, but is not limited to, clustering algorithms. Reinforcement Learning will usually use to type of neural network algorithm.
4. The uses of machine learning are nearly limitless. Although I listed here as the last step, determining the use case or business application should probably be the first step. For example, we would use logistic regression to determine whether a house would sell at a certain price or not and we would use linear regression to predict the future price of a house. You should keep in mind that machine learning business applications typically require the use of more than one machine learning type as well as multiple algorithms.
I hope this sketch serves as a useful guide. Feel free to share and use as needed.
Thoughts and comments as well as suggestions for other sketched explanations are welcomed.
Notebook Thoughts: Google vs Facebook Ecommerce Solutions
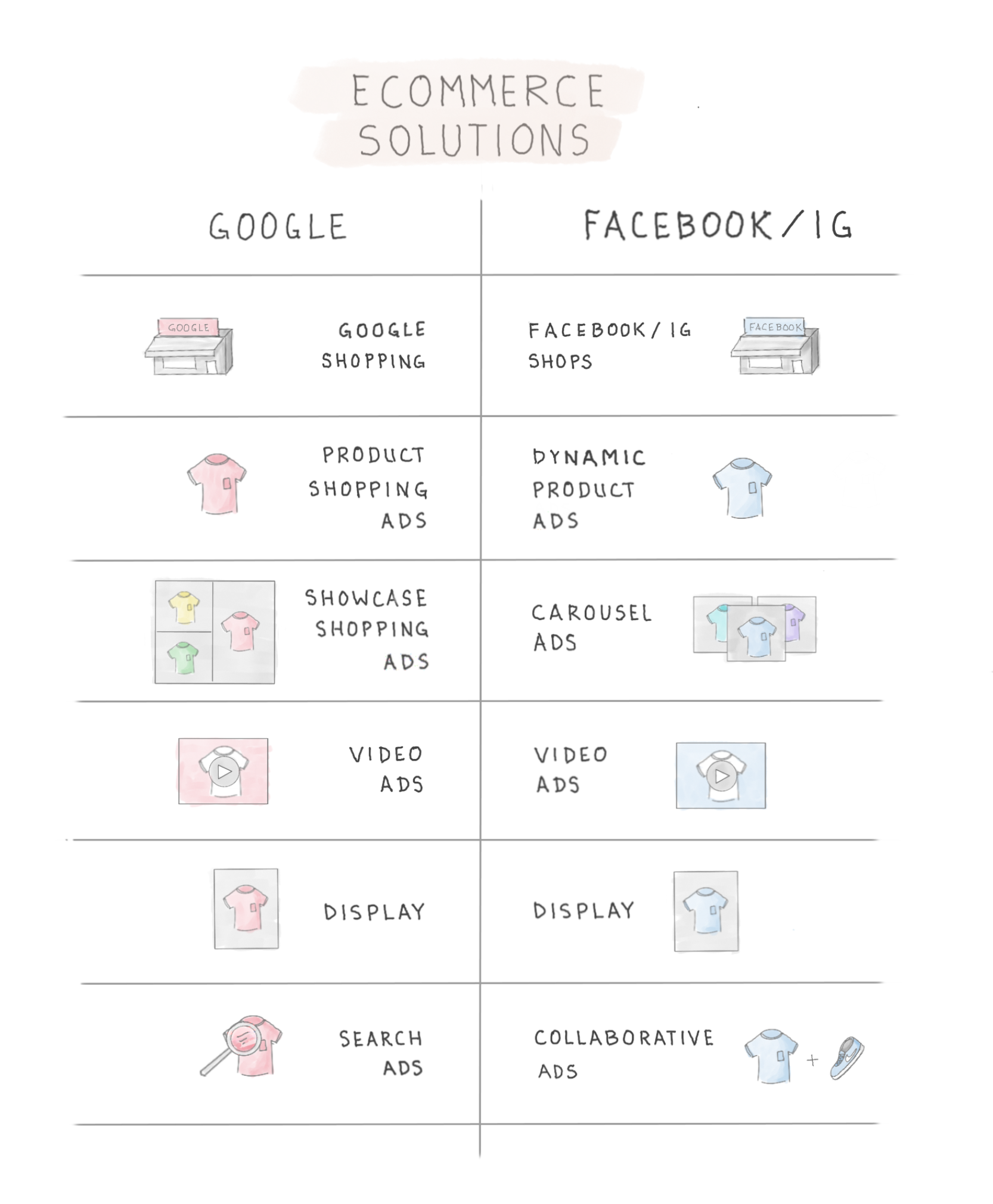
This is simple guide of ecommerce advertising solutions offered by Google and Facebook / Instagram. There is, of course, a lot more to it than the brief descriptions provided below.
SHOPS: Googles Shopping is basically a shopping channel where products are searchable but transactions occur at the brand’s website. IG/FB shops are online stores where transactions can take place within the app or at the brand’s website
PRODUCT LISTINGS: Google Shopping Ads, also known as Product Listing Ads, are single product ad units. Facebook’s equivalent are the Dynamic Product Ads.
MULTI-PRODUCT LISTINGS: Showcase Shopping Ads and Carousel Ads are Google and Facebook’s respective product ad units that allows for the listing of multiple related or unrelated products.
VIDEO ADS: Video Ads on Google can be served on YouTube or across the Google Display Network. Formats vary and can include live-streaming. FB/IG’s video ads can appear in Feed or Stories and can also include live-streaming.
DISPLAY ADS: Google’s display ads can be served across the Google Display Network. Similar to video, FB/IG’s display ads can appear in Feed or Stories as well as through the Facebook Audience Network.
SEARCH ADS: Google Search Ads are ad units that appear alongside search results based on relevant keywords. They can include ad extensions which allow the brand to include product or business details.
COLLABORATIVE ADS: Facebook Collaborative Ads allow brands that sell products through retailers and merchants to run direct sales campaigns
Notebook Thoughts: Understanding Headless Commerce
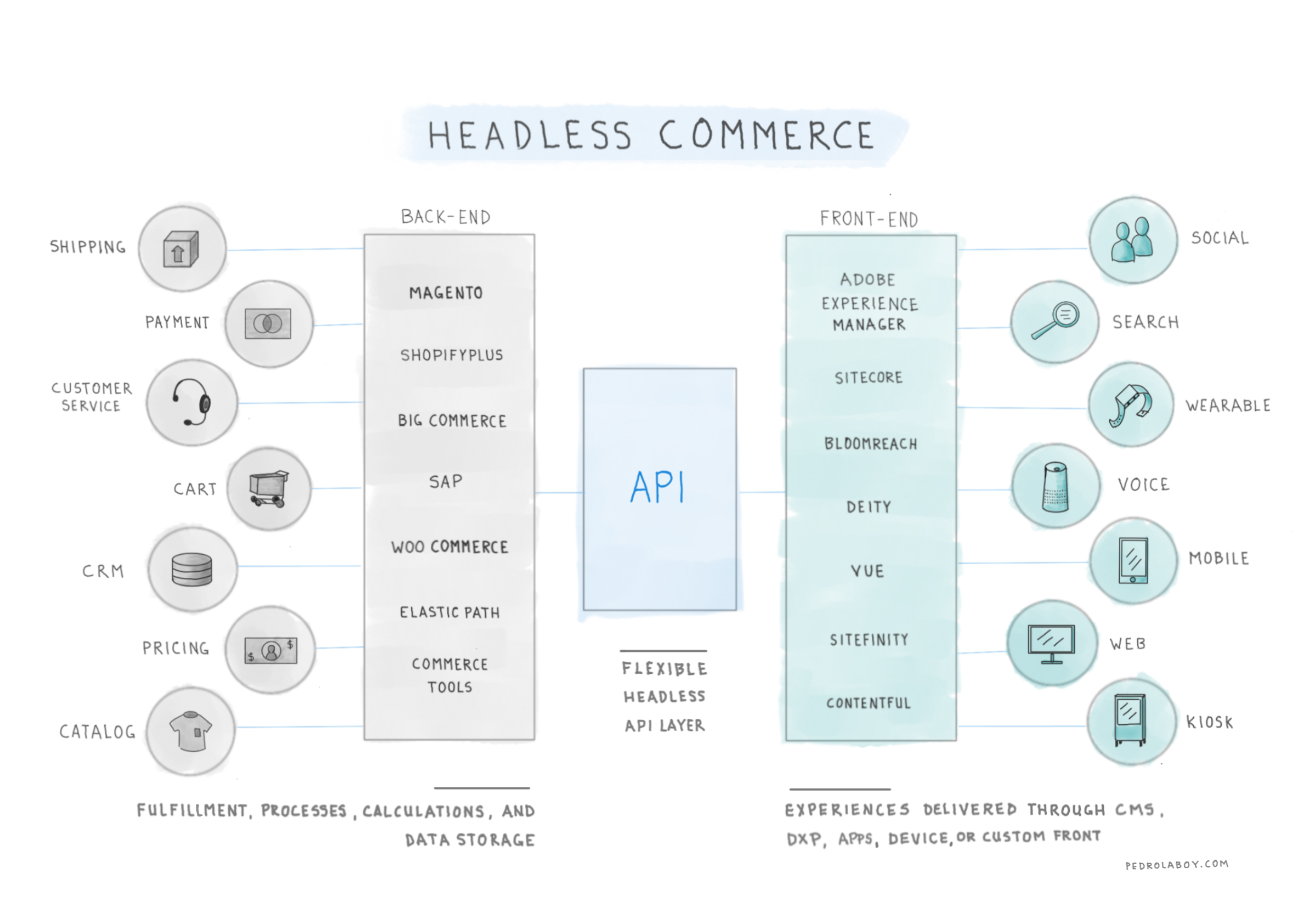
Headless commerce decouples back-end platforms responsible for fulfillment, operations, processes, calculations and data from front-ed platforms responsible for delivering experiences through technologies such as CMS (content management systems), DXPs (digital experience platforms), PWAs (progressive web applications) or custom solutions. One can say that the back-end is “headless” and front-end offers multiple “heads.” This is made possible through the use of a flexible API layer
Notebook Thoughts: Media Agencies’ Ansoff Matrix
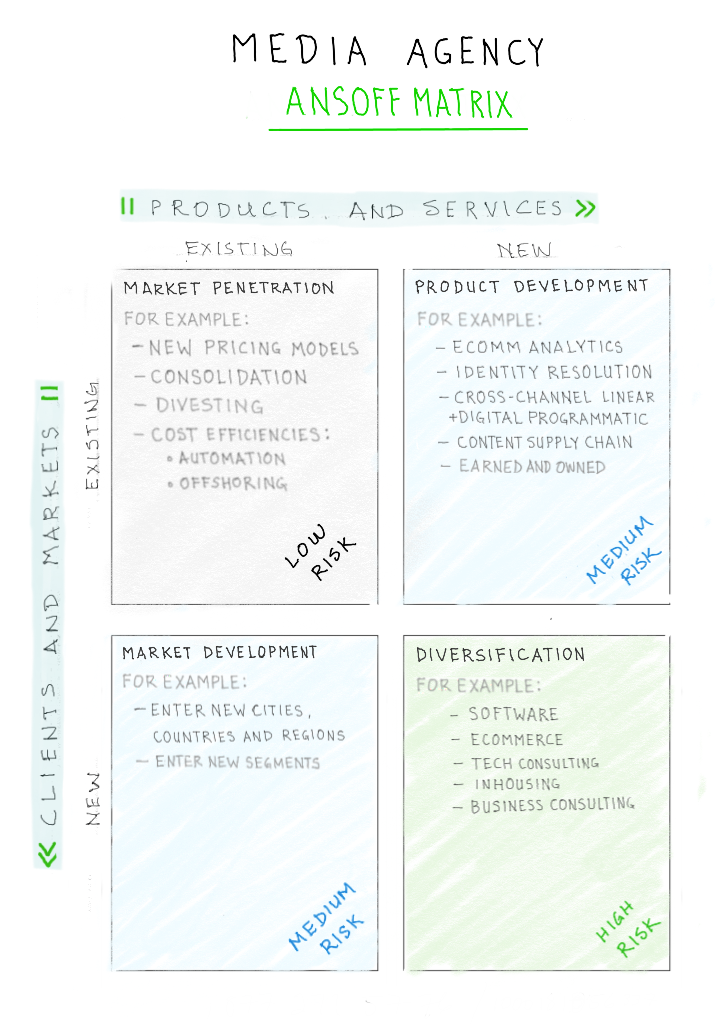
Recently, I was having a conversation with a friend about strategic choices and risks in our industry. The best way to map the two is to think of them in terms of the Ansoff Matrix. Ansoff argued that business growth can only come from two sources: new products and services or/and new clients and markets.
Market Penetration – One can grow existing clients or win new clients in your current market by offering a better value propositions. These could include new pricing models, efficiencies through consolidation or lower costs through off-shoring or automation. This is considered a low risk strategy.
Product Development – One can create new products and services that complement the existing suite. These could include ecommerce analytics, identity resolution, digital/linear cross-channel programmatic or new products around content creation and management. This is considered a medium risk strategy.
Market Development – This one is straight forward. Grow business my entering new markets with existing and/or new products and services. This is considered a medium risk strategy.
Diversification – One can develop brand new products and services that are outside the current areas of expertise. This could include tech consulting, software development, ecommerce management, or in-housing solutions (within expertise but can cannibalize existing services). This is considered a high risk strategy.
Notebook Thoughts: Understanding Marketing Attribution
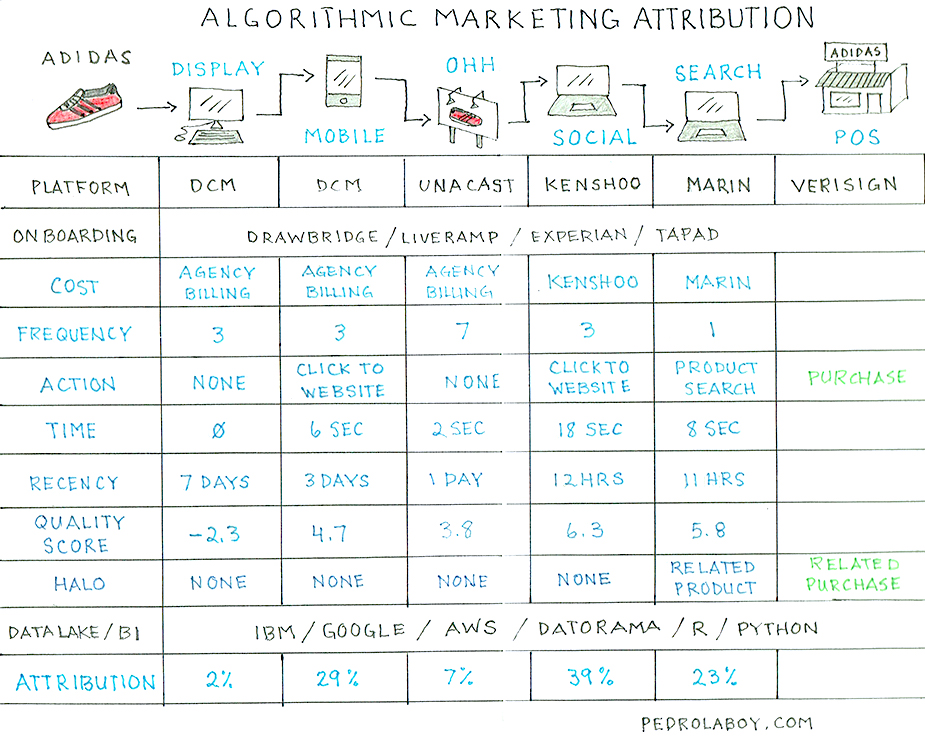
Algorithmic or Probabilistic Attribution uses statistics and machine learning to determine the probability of conversion across marketing touchpoints. In other words, how much of a conversion should be attributed to each channel. In order to keep things simple, I randomly chose a few variables—out of dozens or more—that could go into our model. Let’s go through the rows one at a time.
Touchpoints – This is a mix that includes online as well as offline touchpoints.
Platforms – These are some of the platforms that can be used to collect the data for each touchpoint.
Cost – Cost is one of our most important variables since it helps us determine ROI. Just because something is effective does not meant it is efficient.
Frequency – How many times was our ad/content served to the prospect.
Action – Did the prospect take any action upon viewing our content (i.e. click on it, etc.).
Duration – What is the duration of an engagement? In this example, our prospect spent 6 seconds on landing page after clicking on a mobile ad. Also, we used technology to determine that the prospect looked at an OHH ad for 2 seconds.
Recency – When was the last engagement before conversation. The closer the recency between touch points the higher the weight to the precedent one. Here we see that the prospect conducted a product search within the hour after he/she engaged a social ad/content. Thus, “Social” would get a larger attribution.
Quality score – This a variable that you don’t see often that is extremely important. What is the quality of the ad/content? Was the ad place next to undesirable content? Was the engagement likely from a bot?
Halo – Was there a “halo effect?” That is, did the prospect take a secondary action of value to the brand. In this example, the prospect did a search for a related product and ended up buying that product in addition to the one advertised.
Data Lake / BI – This is the infrastructure needed to process the data and run the machine learning models.
Attribution – Self-explanatory.
Notebook Thoughts: A Data Science Approach to Marketing
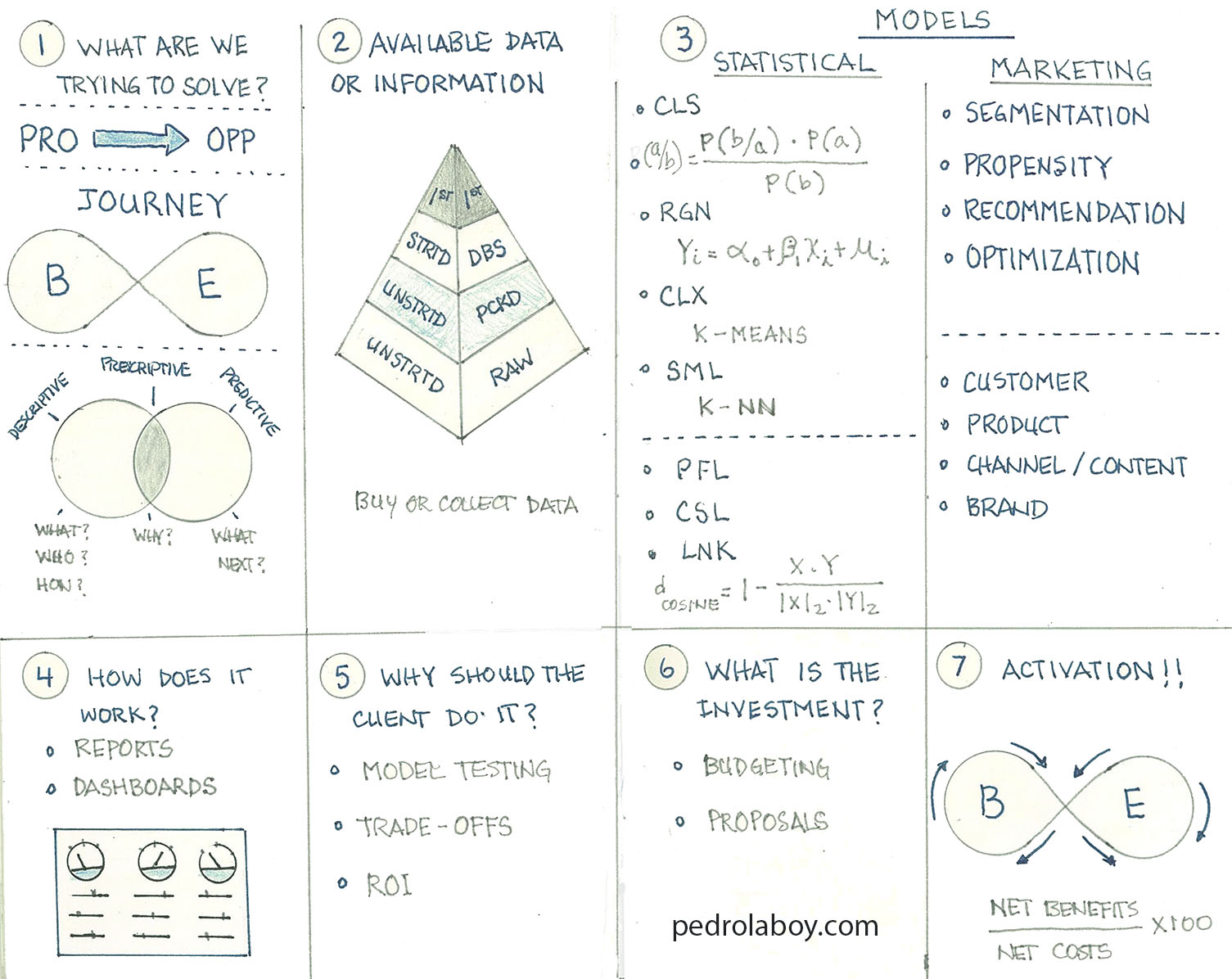
- Why, how, who and what are we trying to solve for? Are we solving a problem or seizing an opportunity? How does it align to the consumer journey?
- Do we have the required data? Are we buying or collecting? Is it structured or unstructured? What about variety, velocity, veracity and volume of data?
- What are the appropriate statistical model? Classification, regression, clustering, similarity, etc.? What about marketing models? Segmentation, propensity, etc.?
- What is the deliverable and how does it work? Are we delivering through dashboards? Will it need to be real time?
- Why should the client move forward with the recommended solution?
- What is the investment required?
- How are we going to test and optimize our proposed solution?
Notebook Thoughts – Building a Loyalty Program Simulator
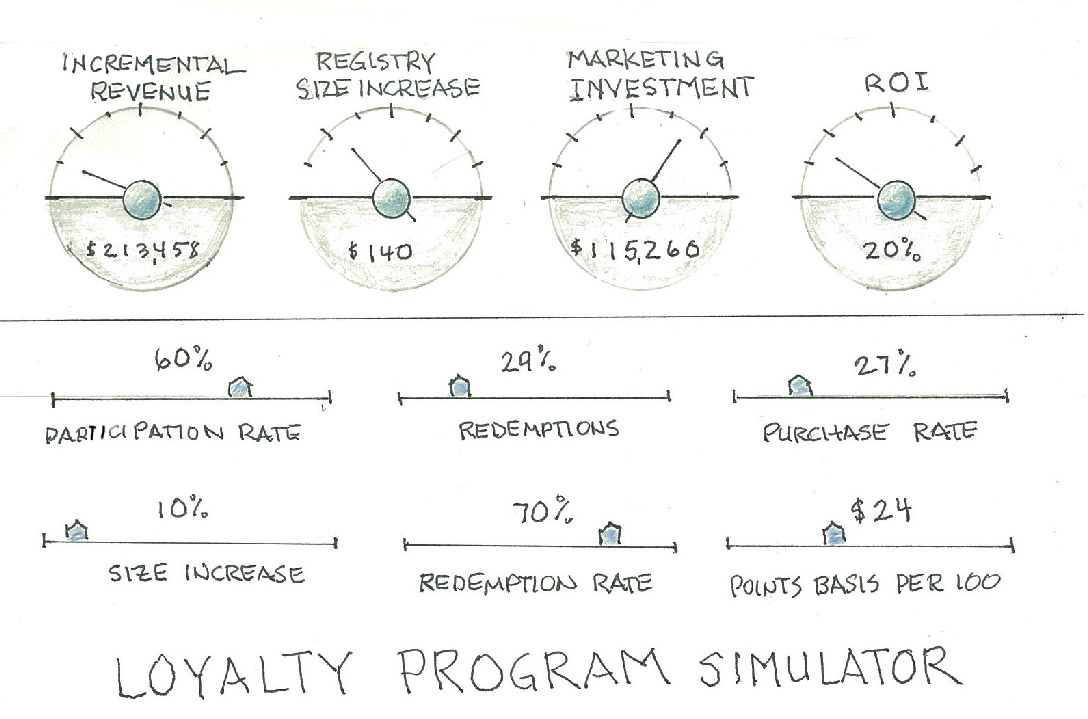
When possible, brands should use simulation to forecast the ROI of marketing programs and initiatives. The above is a sketch/draft of a loyalty program simulator I crafted for one of the largest home furnishings brands in the world.